Unleashing the Power of AI
HPC's computational power enables promising Alzheimer’s research
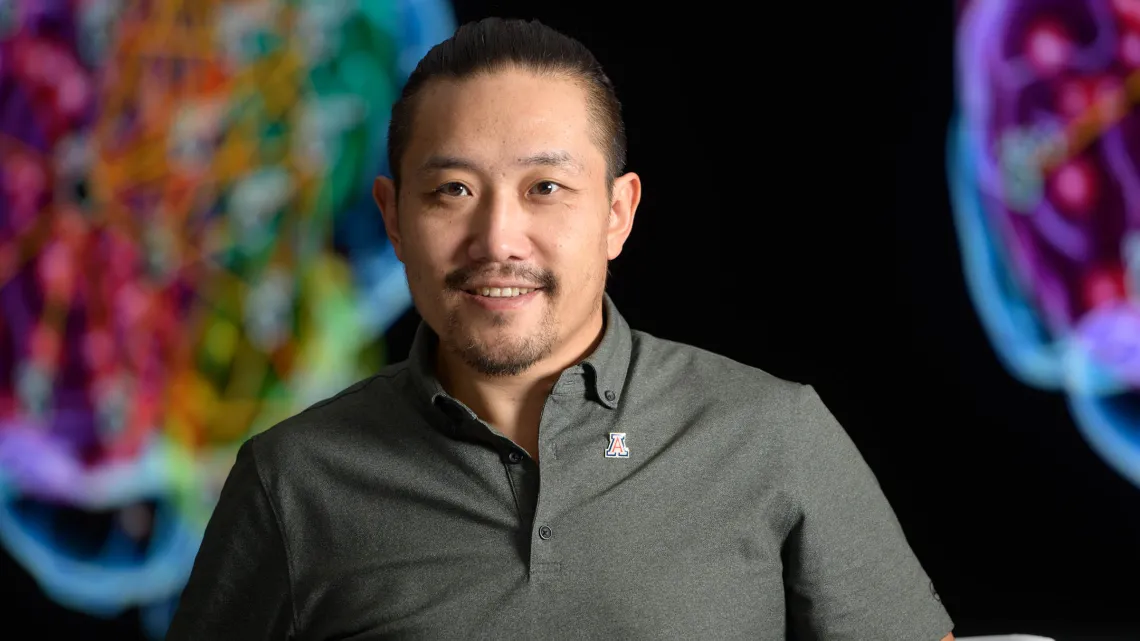
When families face an Alzheimer’s diagnosis, their hopes hinge on the development of drugs that can help.
That wait may be shorter, thanks to remarkable strides in Alzheimer's disease research by Dr. Rui Chang, an Associate Professor of Neurology at the University of Arizona College of Medicine. With a background in computer science, specializing in Artificial Intelligence (AI) and machine learning, Dr. Chang has dedicated over 15 years to developing advanced AI methods to analyze genomic big data of fatal human diseases like Alzheimer’s. Through AI, he is revolutionizing our comprehension of the disease and identifying potential drug targets and treatments.
Dr. Chang's groundbreaking research heavily relies on the High Performance Computing (HPC) resources including their consulting and infrastructure teams. Leveraging the robust capabilities of UITS's HPC systems, these resources empowered Dr. Chang's AI engine to search novel drug targets and treatments by weeding through massive amount of genomics data in human diseases, enabling significant scientific breakthroughs.
Use of the HPC System is available to support University projects. Dr. Chang’s AI platform requires significant computational resources in the HPC system, with each model taking several days to run and consuming hundreds of thousands of CPU hours. To accommodate their complex and resource-intensive models, Dr. Chang’s team received special allocations for their unique requirements, which were approved by the Research Computing Governance Committee (RCGC) which us responsible for overseeing research initiatives.
Creating the Models
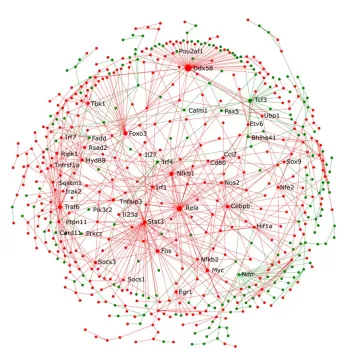
Example of high dimensional network modeling to uncover novel targets for drug development.
Dr. Chang and his team analyzed over 2,000 post-mortem samples of brains affected by Alzheimer's, using AI algorithms to create more than 100 AI models of the Alzheimer’s brain. This approach has accelerated the identification of potential drug targets and streamlined the research process. By leveraging AI's capacity to decipher genetic code, Dr. Chang's team has discovered over 6,000 promising targets and over 3,000 drugs including FDA-approved, natural products and new chemical compounds worth investigating.
The AI algorithm developed by Dr. Chang understands genetic language, much like the more well-known AI programs such as ChatGPT and Midjourney understand human language and visual art, respectively. His team was the first to use AI machine learning to find genetic targets in other conditions such as Lewy body dementia, diabetes and melanoma.
AI algorithms process data using statistical models and computational power to generate new hypothesis. They learn patterns and relationships from labeled data through machine learning, creating a network model. These models find connections and relationships among molecules that are not immediately obvious.
“When you run a machine learning model like this, you really don't know what the outcome is going to be because it is kind of a black box," explained Chris Reidy, Manager of Research Consulting in Research Technologies. "The model will run iterations and produce output. The outcome you get can be surprising because it's just figuring out connections that you don't know exist at the outset."
Benefits of the Research
In a University of Arizona College of Medicine newsroom article, Dr. Chang discussed his application of AI in unraveling the disease.
“We’re hopeful that AI is a game changer,” Dr. Chang said. “In five years, I hope there are more clinical trials for drugs for treatment of Alzheimer’s disease, and in 10 years, I hope there will be a couple of FDA-approved drugs to stop or potentially reverse disease progression.”
The integration of AI has not only expedited the identification of drug targets but also enabled the screening of millions of compounds against thousands of targets. This high computational power of the HPC system narrows down the pool of drug candidates, paving the way for clinical trials on the most promising compounds. Dr. Chang's innovative approach, combining AI, genomic data, and computational modeling, holds great promise for developing effective Alzheimer's treatments faster.